Confusion Matrix:
Consider a binary classification problem where we aim to predict whether an email is spam or not spam. The confusion matrix for this scenario is structured as follows:
| Predicted Not Spam | Predicted Spam |
---------------------|---------------------|-----------------|
Actual Not Spam | TN | FP |
---------------------|---------------------|-----------------|
Actual Spam | FN | TP |
Here:
- TN (True Negative): Emails correctly predicted as not spam.
- FP (False Positive): Emails incorrectly predicted as spam (Type I error).
- FN (False Negative): Emails incorrectly predicted as not spam (Type II error).
- TP (True Positive): Emails correctly predicted as spam.
Accuracy:
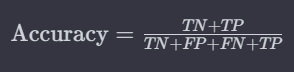
Accuracy measures the overall correctness of the model, providing the ratio of correctly predicted instances to the total instances.
Precision:
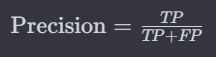
Precision gauges the accuracy of positive predictions, answering the question: Of the instances predicted as positive, how many are truly positive?
Recall (Sensitivity or True Positive Rate):
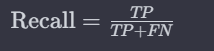
Recall assesses the model’s ability to capture all positive instances, answering: Of all actual positive instances, how many were predicted correctly?
F1 Score:

The F1 score, being the harmonic mean of precision and recall, provides a balanced measure between the two metrics. These evaluation metrics collectively offer a comprehensive assessment of a model’s performance, crucial in scenarios with imbalanced classes.